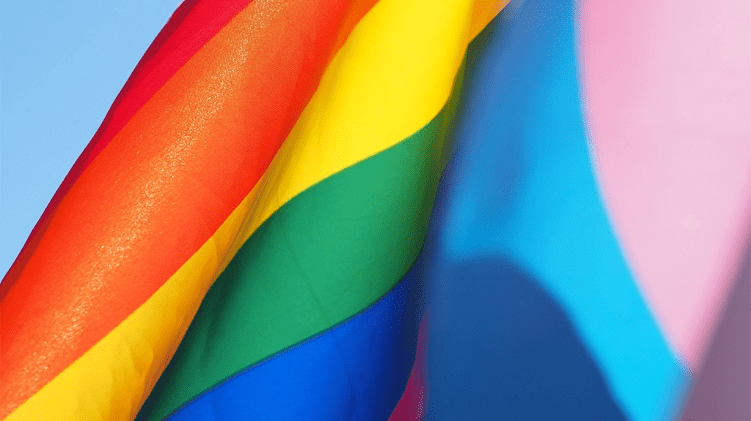
The thrill over generative AI—and AI in general—has reached the multi-trillion-dollar healthcare industry, caused by the news of ChatGPT handing the United States Medical Licensing Exam (USMLE) and the quick introduction of new healthcare-related AI applications. Bill Gates, for instance, recommends using generative AI tools for immediate diagnoses of patients. While believing that AI will inevitably misdiagnose patients, Gates asserts that the upside is worth the trouble.
Averting misdiagnoses that can impact, at the very least, a patient’s quality of life relies a lot on the quality and accessibility of the health data fed into the AI prototype. The current excitement notwithstanding, the evolution of AI healthcare solutions has been severely restrained by the absence of comprehensive and suggestive real-world health data.
“So much of healthcare today carries data very close, it’s very challenging,” states Dr. John Halamka, President of Mayo Clinic Platform. In a paper printed last year, Halamka and other researchers discussed the significance of high-quality data for the successful undertaking of healthcare AI: “…even robustly built models employing state-of-the-art algorithms may fail once tested in realistic surroundings due to unpredictability of real-world conditions, out-of-dataset systems, characteristics of deployment infrastructure, and absence of added value to clinical workflows relative to price and potential clinical troubles.”
Mayo Clinic’s answer to the health data challenge and testing AI solutions in real-world healthcare was to designate a data platform three years ago, based originally on the de-identified records of 10 million patients. For reasonable measure, the Mayo Clinic Platform also contains:
“In completing this entire corpus of multimodal, de-identified data, we’ve helped over 150 organizations to come in to develop and validate AI standards,” states Dr. Halamka.
One of these associations is Atropos Health, offering physicians detailed and correct consultation and possible answers to even the most mysterious medical questions. This “digital consultant” may even locate and suggest emergent treatments. It began as a research project at Stanford University, pursuing the belief that “you should be able to press a button and have the patient in front of you studied against every patient of the past and be informed, well, you better believe this, that, or the other disease,” expresses Dr. Halamka.
Today, operating its proprietary search and analysis programs, Atropos Health’s AI tool causes an automated report, a Prognostogram, in reply to a physician inquiry, assisting in medical decision-making. While it normally takes weeks to determine the best course of remedy for an individual with a challenging case, a Prognostogram presents clinicians’ actions, with evidence, sometimes.
When Mayo Clinic and Atropos Health declared their partnership last November, Brigham Hyde, CEO of Atropos Health, explained its anticipated benefits: “The worth of real-world evidence at Mayo Clinic would construct the Prognostogram more efficient at advising doctors as they deliver care. In turn, this could authorize Mayo Clinic to make new discoveries, develop new treatments, and enhance patient health all over the world.”
A review of the responses by the Atropos’ technology to the primary 100 requests from its usefulness concluded that “on-demand evidence years to inform clinical decision-making is an achievable idea,” and that “as extensive patient data repositories are created, the potential to profit from such a service is tremendous.”
The Mayo Clinic data storage provides high-quality, exhaustive, and longitudinal clinical data. It is incrementally refreshed as more data is added to patients’ medical documents, giving users access to continually growing data with near real-time data.
The data, nevertheless, was initially limited to patients’ background with the Mayo Clinic. Last July, the Mayo Clinic Platform expanded its reach by collaborating with Mercy, one of the 25 largest U.S. health strategies. The combination of Mercy’s different populations and geographic areas (with its 500 million de-identified patient encounters) and Mayo is anticipated to improve accuracy, reduce model bias and complete more diverse, and therefore more vital, therapy guidance for patients.
“This is what we dub a distributed data network of bringing associations around the world together so that privacy-protected model growth and validation can happen at multiple institutions. This gets us to a more reasonable state as opposed to locking our data in silos, as has been so much of the past,” states Dr. Halamka.
“A few specialties have changed since then…” wryly notes Dr. Halamka, who earned his medical degree 30 years ago, observing that “50% of what I understood in medical school is inaccurate. I just don’t know which 50%.” This is probably true—or perhaps even more so—for physicians that got their degrees more newly. “So what AI can do for me is it can digest the corpus of the publications as well as the multimodal patient data and say, John, regard these five diagnoses,” says Halamka.
But he warns the productive AI enthusiasts that turning the decision-making completely over to a statistical model is fraught with dangerous prospects. “I don’t think AI means ‘artificial intelligence,’” voices Dr. Halamka. “AI implies augmented intelligence.”